Understanding the Real Cost of GenAI Webinar – Our Key Takeaways
Date Published
Categories
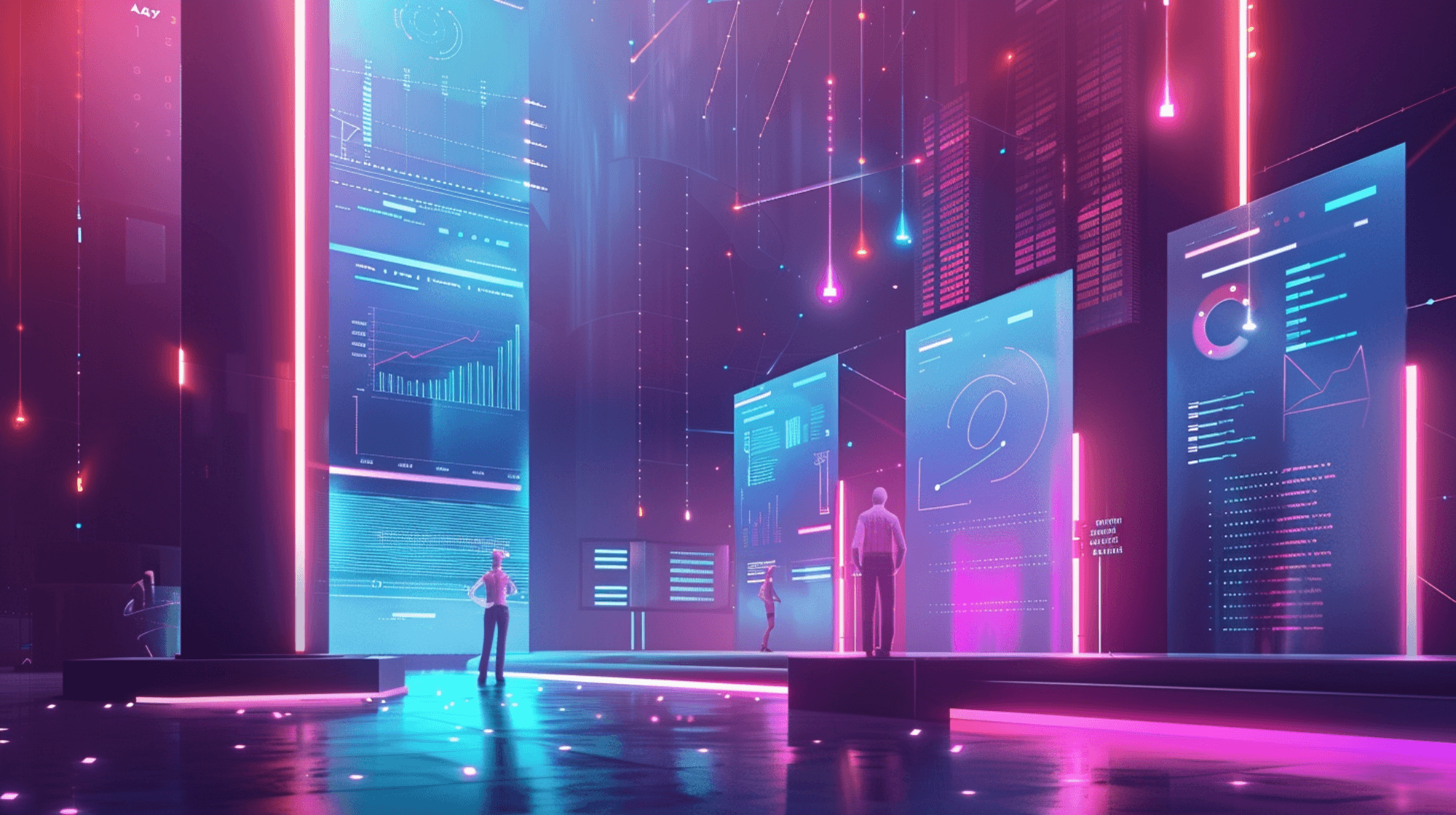
In our Understanding the real cost of GenAI webinar, we provided a detailed overview of the key cost drivers behind GenAI solutions and how the principles and practices from FinOps could be applied to AI technologies, to help you understand, manage and optimise your investment.
Below is a summary of the key points raised:
Key takeaways:
FinOps for AI builds upon the FinOps framework with a focus on maximising the value from AI technology. It aims to give visibility as to how and where AI is being consumed within an organisation, to provide timely and accurate data that aids decisions on how to optimise the investment.
Cost Transparency will be key to managing your GenAI investments.
- What data will you need?
Usage metrics will be key, so using Cloud Service Provider’s APIs to pull in usage data will be critical - As part of understanding the usage and costs data, we need to understand the different cost levers and ensure we have the correct information for calculating costs before we can begin to paint the full picture
- The new FOCUS™ framework provides a standardised billing file, allowing us to see easier than ever what data is available to us from the native billing file
- Challenges? Existing reporting and tooling may not be linked to the required data sources, so a transformation effort may be required to allow teams to build out the correct reporting.
Cost Drivers: operational expenses of AI can quickly exceed expectations, They are usually driven by factors like inappropriate model selection, inefficient workload design, and uncontrolled token usage.
Models
- Choose a model that fits the specific needs of your workload. A smaller, cheaper model could be sufficient for the task - don’t just go for the default option.
- Be aware of Legacy Models. AI moves quickly. Sleeping on models can mean costs spiral. When newer, more powerful models are released, vendors introduce pricing to encourage applications to move to newer models, freeing up capacity.
Token Consumption
- Model pricing is based on the input and output tokens consumed during model interactions.
- Input token counts can quickly spiral out of control due to inefficient prompts and context injection.
Look at ways to optimise the prompt and context to reduce the total tokens used for inputs. For example, simplify your prompt by removing unnecessary information and detail system descriptions.
Pricing Models
- The most common pricing utilised is Pay as you go, as it offers flexibility and scalability for workloads.
- Once a pattern for usage has been established, it is worth reviewing some of the other pricing options available.
- PTUs (Provisioned Throughput Units) allow you to commit to monthly and yearly reservation purchases, providing a discount on the standard pricing, which can offer a considerable saving for those with workloads that have high token consumption.
- Some PTUs also offer a token limit. This means there is no danger of fluctuating costs outside of the price paid if demand increases.
Indirect Costs
- Be aware of the other costs associated with a GenAI solution. A model is just a single part of the application, and many rely on other infrastructure to be able to fulfil their task.
- For example, Storage. A solution might require a database to supply the contextual data needed as part of an input prompt. Are there opportunities to optimise the database engine used to store this information required by the AI model?
- Similarly, take into consideration the Compute costs. If we want to fine tune a model, what are the compute requirements, and should we attribute this as a cost linked to the AI capability, rather than categorising as an independent compute cost?
In the coming weeks, we will be publishing articles to look at each cost driver individually and how to optimise it, as well as tips on how to adapt your FinOps practices to successfully manage your GenAI solutions.
If you have any questions in the meantime, or would like our help with your GenAI solution, please feel free to message directly.
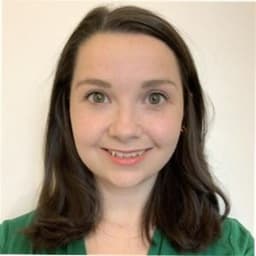
Alice Keal
Alice is a Senior Delivery Consultant at Cortex Reply and leads their FinOps offering, supporting businesses to understand and manage the costs associated with their technology investments.
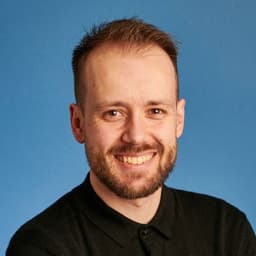
Andy Payne
Andy is a Senior AI Solutions Engineer, specialising in the enablement and development of AI applications within public cloud environments. He holds a BSc in Computer Science and has experience working across multi-cloud environments in regulated industries